3D Biophysical Modeling
Overview
Dr. Brian Bailey is leading the development of biophysical modeling tools that will complement and enhance the AI-enabled sensing portion of GEMINI's mission, as well as enable prediction of genotype by environment interactions. The models are meant to more accurately extract traits from sensor measurements as well as predict how changing climate conditions will affect crops. The modeling will also help predict which traits crops will need to adapt to a rapidly changing climate.
Helios 3D Plant Modeling Framework
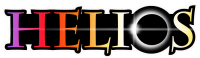
The Helios 3D simulation framework (Bailey 2019) enables fully resolved simulation of plant biophysical processes across scales ranging from leaves to whole-canopies.
Synthetic (Simulated) Sensor Data for Improvement of Machine Learning Based Phenotyping
In order to enhance phenotyping, a “syn to real” approach is used where synthetic images of plants are created across various environments and growth stages. These images can then be used to help train AI models by providing a diverse range of data, which can be supplemented with real images when necessary. This approach eliminates the need for extensive manual labeling, saving time and enabling faster model development for traits that are otherwise difficult to label.
The synthetic data can be used to model complex interactions such as radiation transfer across different plant materials, allowing for the simulation of multispectral images that incorporate underlying biophysical processes. These models can be applied to a wide range of traits and environments enabling better predictions for plant performance, for example, integrative traits like leaf angle, which is influenced by multiple factors such as leaf area distribution, photosynthetic capacity, and environmental conditions. The aim is to provide a more comprehensive understanding of plant performance by combining these different data sources.
Predicting Plant-Environment Interactions
Plant structural and functional traits interact to determine how plants interact with the environment. Predicting future performance involves parameterizing 3D models from sensing data to make predictions about how plants will perform under different environmental conditions. For instance, phenotyping water use-related traits by analyzing canopy temperature measurements to assess crop water stress. Dr. Bailey’s team has successfully developed models that extract parameters for stomatal conductance, a key factor in understanding how plants regulate water use, which once trained with synthetic data, can be applied to real field data to predict plant performance in various environments including those impacted by climate change. They have also developed a generalized plant architecture model that allows for consistent parameterization across different species, facilitating broader application of these techniques in crop research.